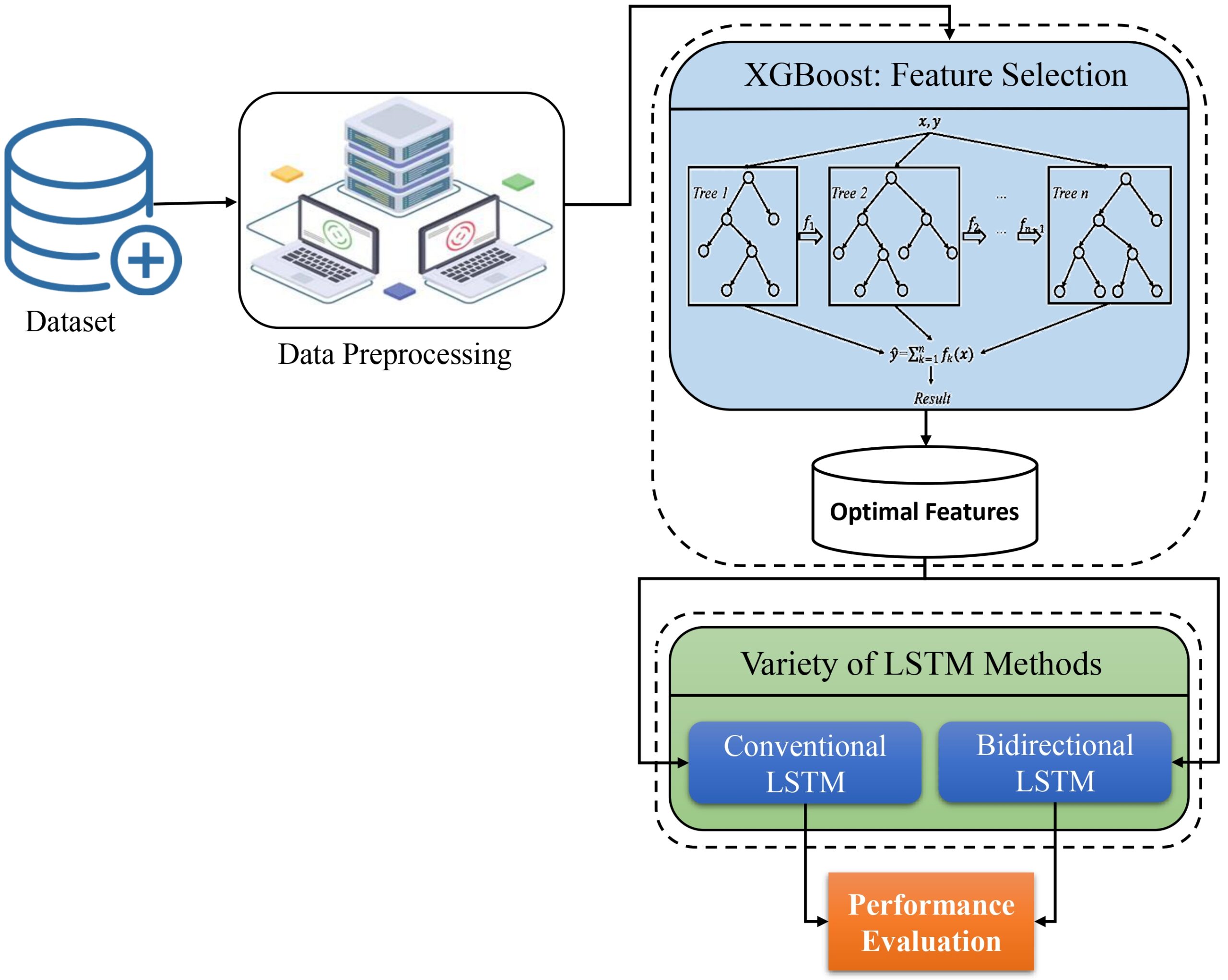
Predictive Power of XGBoost_BiLSTM Model: A Machine-LearningApproach for Accurate Sleep Apnea Detection Using Electronic HealthData
- Post by: Ashir Javeed
- 18 December, 2023
- No Comment
Abstract
Sleep apnea is a common disorder that can cause pauses in breathing and can last from a few seconds to several minutes, as well as shallow breathing or complete cessation of breathing. Obstructive sleep apnea is strongly associated with the risk of developing several heart diseases, including coronary heart disease, heart attack, heart failure, and stroke. In addition, obstructive sleep apnea increases the risk of developing irregular heartbeats (arrhythmias), which can lead to low blood pressure. To prevent these conditions, this study presents a novel machine-learning (ML) model for predicting sleep apnea based on electronic health data that provides accurate predictions and helps in identifying the risk factors that contribute to
the development of sleep apnea. The dataset used in the study includes 75 features and 10,765 samples from the Swedish National Study on Aging and Care (SNAC). The proposed model is based on two modules: the XGBoost module assesses the most important features from feature space, while the Bidirectional Long Short-Term Memory Networks (BiLSTM) module classifies the probability of sleep apnea. Using a cross-validation scheme, the proposed XGBoost_BiLSTM algorithm achieves an accuracy of 97% while using only the six most significant features from the dataset. The model’s performance
is also compared with conventional long-short-term memory networks (LSTM) and other state-of-the-art ML models. The results of the study suggest that the proposed model improved the diagnosis and treatment of sleep apnea by identifying the risk factors.
Keywords: Sleep apnea · Deep learning · Computer vision · Feature engineering
https://doi.org/10.1007/s44196-023-00362-y
Reference
https://link.springer.com/article/10.1007/s44196-023-00362-y