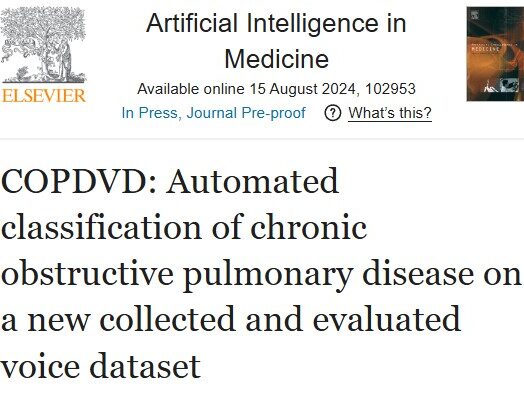
COPDVD: Automated classification of chronic obstructive pulmonary disease on a new collected and evaluated voice dataset
- Post by: Alper Idrisoglu
- 23 August, 2024
- No Comment
Abstract
Background: Chronic obstructive pulmonary disease (COPD) is a severe condition affecting
millions worldwide, leading to numerous annual deaths. The absence of significant symptoms
in its early stages promotes high underdiagnosis rates for the affected people. Besides
pulmonary function failure, another harmful problem of COPD is the systemic effects,
e.g., heart failure or voice distortion. However, the systemic effects of COPD might provide
valuable information for early detection. In other words, symptoms caused by systemic
effects could be helpful to detect the condition in its early stages.
Objective:The proposed study aims to explore whether the voice features extracted from the
vowel “a” utterance carry any information that can be predictive of COPD by employing
Machine Learning (ML) on a newly collected voice dataset.
Methods: Forty-eight participants were recruited from the pool of research clinic visitors at
Blekinge Institute of Technology (BTH) in Sweden between January 2022 and May 2023. A
dataset consisting of 1246 recordings from 48 participants was gathered. The collection of
voice recordings containing the vowel “a” utterance commenced following an information
and consent meeting with each participant using the VoiceDiagnistic application. The
collected voice data was subjected to silence segment removal, feature extraction of baseline
acoustic features, and Mel Frequency Cepstrum Coefficients (MFCC). Sociodemographic
data was also collected from the participants. Three ML models were investigated for the
binary classification of COPD and healthy controls: Random Forest (RF), Support Vector
Machine (SVM), and CatBoost (CB). A nested k-fold cross-validation approach was
employed. Additionally, the hyperparameters were optimized using grid-search on each ML
model. For best performance assessment, accuracy, F1-score, precision, and recall metrics
were computed. Afterward, we further examined the best classifier by utilizing the Area
Under the Curve (AUC), Average Precision (AP), and SHapley Additive exPlanations
(SHAP) feature-importance measures.
Results: The classifiers RF, SVM, and CB achieved a maximum accuracy of 77%, 69%, and
78% on the test set and 93%, 78% and 97% on the validation set, respectively. The CB
classifier outperformed RF and SVM. After further investigation of the best-performing
classifier, CB demonstrated the highest performance, producing an AUC of 82% and AP of
76%. In addition to age and gender, the mean values of baseline acoustic and MFCC features
demonstrate high importance and deterministic characteristics for classification performance
in both test and validation sets, though in varied order.
Conclusions: This study concludes that the utterance of vowel “a” recordings contain
information that can be captured by the CatBoost classifier with high accuracy for the
classification of COPD. Additionally, baseline acoustic and MFCC features, in conjunction
with age and gender information, can be employed for classification purposes and benefit
healthcare for decision support in COPD diagnosis.
Keywords
Acoustic features; Mel Frequency Cepstrum Coefficients; Automated
classification; Chronic Obstructive pulmonary disease; Machine Learning.
Reference
Idrisoglu, A., Dallora, A. L., Cheddad, A., Anderberg, P., Jakobsson, A., & Berglund, J. S. (2024). COPDVD: Automated classification of chronic obstructive pulmonary disease on a new collected and evaluated voice dataset. Artificial Intelligence in Medicine, 102953.
Link
DOI: doi.org/10.1016/j.artmed.2024.102953